Ongoing Projects
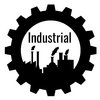
Cool Street Project
Total Duration: 2 M.Sc. for 1 year
The CoolStreet project collaborating with ClimateFlux GmbH. aims to develop a service platform in the format of an API to generate a map-based application that displays the temporal-spatial distribution of urban heat to allows pedestrians and cyclists to choose alternative routes to minimize their exposure to heat stress. Unlike conventional routing applications mainly focusing on travel distance and time, this project addresses the environmental influences on pedestrians and cyclists, promotes thermal comfort routes and aims to improve the awareness of city climate. In this project, we will adopt predictive computer modeling methods (AI) and data-driven approaches to predict micro-scale environmental exposure levels. The ML algorithm is trained on a large amount of building and tree height models to generate hourly shadow distribution and an integrated urban comfort index will be formed and applied as one of the weighting attributes of road segment to identify healthier routes in road network of Munich city. A user-centered interface will be designed to support the customized navigation services.

Dense and Deep Geographic Virtual Knowledge Graphs for Visual Analysis
Total Duration: 1 doctoral candidate for 3 years
The DFG project has the goal to effectively integrate and analyze heterogeneous geodata sources by bridging two research fields – Virtual Knowledge Graphs (VKG) and Geovisual Analytics. In the project we will develop on the one hand a set of methodologies and software tools to create densely and deeply linked geospatial knowledge graphs, and on the other hand a set of intuitive visualizations and explainable analytical services over them. The project will provide a complete solution covering the whole life-cycle from the construction and enrichment of GeoVKGs, to their visualization and analysis over them. The developed methodology and software tools will be demonstrated in two real-world use cases. The first one demonstrates climate change in Bavaria, while the second one deals with tourism and mobility data in South Tyrol, managed by NOI Techpark in Bolzano. Although the two use cases have different characteristics, they both represent common and intricate cases of geodata management, and thus require the ability to effectively combine the underlying datasets and to perform complex analytical tasks over them.

OpenStreetMap Boosting using Simulation-Based Remote Sensing Data Fusion - OSMSim
Total Duration: 2 doctoral candidates for 3 years
This DFG project aims to improve building information in OpenStreetMap (OSM) using a simulation-based fusion of heterogeneous remote sensing data and the updated OSM data for follow-up applications. The simulation environment SimGeoI provides the starting point. It is used to compare geometric OSM information with remote sensing data produced under different sensor configurations and at different acquisition times, and to enrich OSM with geometric corrections (position, height) and attributes (e.g. building type, roof structure) gained from a fusion of the different remote sensing data. Three core themes are addressed: First, a methodical framework will be developed, which allows the geometric correction of OpenStreetMap data based on the prediction and comparison of building shapes, using a pair of remote sensing images (optical, SAR or mixed). In a second step, geometrically improved OSM information will be used to extract building-related attributes from multi-modal remote sensing data. Finally, the transferability of the developed methods will be experimentally analyzed and interfaces to follow-up applications will be investigated. The methodology will be accompanied by validation in order to evaluate the positional, thematic and temporal accuracy of derived results.

Guided Unlearning of Cognitive Pitfalls in Georeferenced Social Sensing
Total Duration: 1 postdoc researcher or 1 doctoral candidate for three years
This DFG project addresses bias-induced cognitive pitfalls in social sensing. For selected application scenarios, an interactive platform for guided unlearning of cognitive biases will be developed and prototypically implemented. Unlearning is a radical method of learning. Unlike conventional learning or knowledge accumulation, which is based on the addition of what is new to the learner, an unlearning process is based on the conscious subtraction of something undesirable that already exists, either innately or learned. The project has three objectives: to improve the transparency with regard to the value chain of georeferenced social sensing; to support users’ holistic understanding of cognitive biases in georeferenced social sensing; and to assess users’ capacity of critical reasoning after the training of guided unlearning. A number of guided unlearning experiments will be designed and implemented with biased data for selected real-world scenarios. We will collect preliminary findings and/or raise new questions, relying on two kinds of comparison: between untrained user solutions and benchmark solutions, and between untrained and trained user solutions.
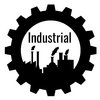
Data-driven exploration and explanation of ethics cases in business world
Begin: since 2021.09
Contact: Chuan Chen / Mengyi Wei
This project funded by industry partner addresses the ethical issues in business world from a data scientific perspective. The ethics cases in big data are mainly reflected in narratives of conflicts, negotiation contents, trial processes and results, professional comments, public opinions, analytical judgments, and constantly updated lists of "should do" and "should not do". However, without AI support, the human brain alone cannot see through the intricate and varying strengths and weaknesses of connections among the elements of a case or among different cases. In this project we attempt to develop an interactive visual analytical platform that can combine the data-driven deep learning ability with knowledge-driven human's reasoning and interpretation ability. The platform will enable us to perform tasks along a value chain such as automatic collection of ethics cases from the globally accessible public media and social media, analysis and clustering of collected data, creation of a specific ontology and knowledge graph, and visual explanation of the knowledge graph. Being driven by big data, the platform may serve the general objectives for different stakeholders in the business world.
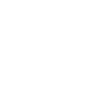
Situated Geovisualization Based on Mixed Reality
Begin: since 2020.12
Contact: Shengkai Wang
Limited spatial information obtained from the real world leads to poor human spatial memory and navigation performance. Mixed reality (MR) enriches accessible information and improves the interaction experience of users' surroundings, reflecting great potential in improving human spatial cognition in recognizing, understanding, memorizing, retrieving, and representing. However, factors like spatial scale and information complexity may have impacts on the usability of MR-based visualizations and thus cause distortion and loss of spatial memory. This project addresses the human spatial cognition issues from the perspective of visualization based on MR. Our research is to explore the behaviors of users, uncover the impacts of visual and spatial factors on usability, and develop an MR-based visualization and visual analysis platform for improving human spatial cognition.
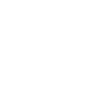
Spatial association and GeoAI
Begin: since 2020.10
Contact: Peng Luo
This project aims to deepen our understanding of spatial association and develop the new Geospatial Artificial Intelligence model (GeoAI). First, this project explores a new way to understand spatial association apart from spatial heterogeneity and spatial autocorrelation. Second, this project attempt to introduce a new understanding of spatial association into AI algorithms. The developed new GeoAI models will be applied to urban computing, social sensing, spatial optimization, population mapping, and other fields.
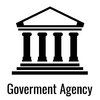
A climate event portal for knowledge discovery
Begin: since 2018.5
Contact: Liqiu Meng, Andreas Divanis
Partners: Bayerische Klimaforschungsnetzwerk (BayKlif): Prof. Dr. Annette Menzel, Technische Universität München, Prof. Dr. Dieter Kranzlmüller, Leibniz-Rechenzentrum, Prof. Dr. Susanne Jochner-Oette, Katholische Universität Eichstätt-Ingolstadt, Prof. Dr. Jörg Ewald, Hochschule Weihenstephan-Triesdorf, Prof. Dr. Wolfgang W. Weisser, Technische Universität München, Prof. Dr. Ulrike Ohl, Universität Augsburg, Prof. Dr. Arne Dittmer, Universität Regensburg, Prof. Dr. Henrike Rau, Ludwig-Maximilians-Universität München
The PhD or post doc researcher will be working with multidisciplinary teams in a Research Cluster on “Bavarian Synthesis Information Citizen Science Portal for Climate research and Scientific Communication” (BAYSICS - Bayerisches Synthese-Informations-Citizen Science Portal für Klimaforschung und Wissenschaftskommunikation).
Project webpage: https://www.bayklif.de/verbundprojekte/baysics/teilprojekt-3/