EuroCrops
EuroCrops is a dataset for automatic vegetation classification from multi-spectral and multi-temporal satellite data, annotated with official LIPS reporting data from countries of the European Union, curated by the Technical University of Munich and GAF AG. The project is funded by the German Space Agency at DLR on behalf of the BMWI (Federal Ministry for Economic Affairs and Energy). This dataset is publicly available for research causes with the idea in mind to assist in the subsidy control of agricultural self-declarations.
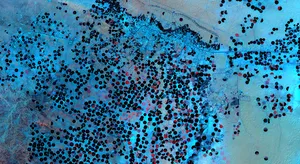
Global Earth Monitor
The Global Earth Monitor (GEM) project is addressing the challenge of continuous monitoring of large areas in a sustainable and cost-effective way. The goal of the project is to establish a new disruptive Earth Observation Data: Exploitation model which will dramatically enhance the utilisation of Copernicus data.
PreTrainAppEO (BMWi/DLR)
The aim of the PreTrainAppEO project is to make the use of AI in the field of Earth observation and remote sensing more attractive and efficient by developing a methodology that uses the approach of pre-trained AI models to achieve generalisability to different standard applications in this field.
Modelling, Prediction and Anomaly detection of Earth Surface Dynamics (TUM IGSSE, Imperial College London)
Deep-learning-based hybrid and uncertainty-aware modelling of the coupled water and carbon cycle with Earth observation data (BMWi/DLR)
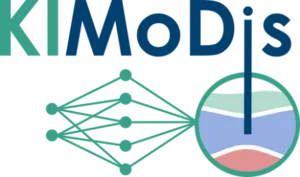
KIMoDIs: Intelligent Monitoring Algorithms for Automated Data evaluation and Plausibility Checks (BMBF)
This sub-project aims to develop innovative data-driven and hybrid modelling approaches that can be used to derive groundwater levels and salinities from earth observation data or to predict them in the form of forecasts. For this purpose, circulation models are also to be created in order to identify and quantify transport processes.
Knowledge Distillation from Big Administrative Data (KnowDisBAD)
The amazing performance of deep learning models ignited the demand for more training data to train better models. An often overlooked data source comes from public service, where significant amounts of administrative data has been collected. These adminstrative data offers a wide variety of knowledge such as argricultural data and parking statistics that can be turned into labels for crop classification and training data for traffic prediction. However a huge challenge in realizing the full potential of administrative data is their inconsistent format which requires data alignment and harmonization. To this end, we propose using large language models to extract information from diverse administrative bodies and create ready to use data.