Ongoing Projects
Horizon Europe - ThinkingEarth
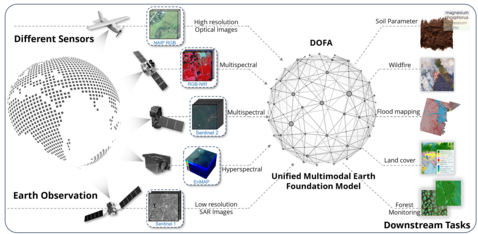
At ThinkingEarth, we view the Earth as a complex unified and interconnected system. To harness the power of Artificial Intelligence (AI), we use cutting-edge techniques, including deep learning, causality, eXplainable AI, and physics-aware Machine Learning. We leverage the predictive abilities of Self-Supervised Learning and Graph Neural Networks to develop task-agnostic Copernicus Foundation Models and a Graph representation model of the Earth.
We demonstrate the potential of these assets through small-scale downstream Spotlight Applications, as well as large-scale use cases that integrate distributed industrial and user non-EO datasets. These use cases address ambitious problems with high socio-environmental impact and new business growth opportunities, such as accelerating Europe's clean energy transition and independence from volatile fossil fuels, understanding Earth's processes by modeling causal Earth system teleconnections, and assessing and modeling the impact of current and future Climate emergency in biodiversity and food security.
International AI4EO Future Lab
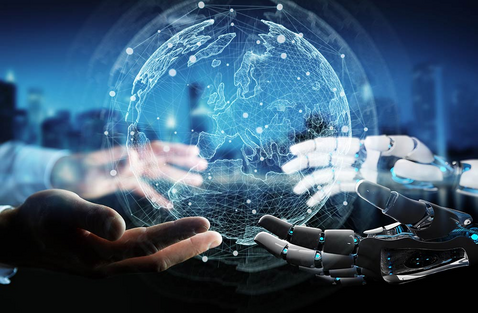
The Future Lab AI4EO (Artificial Intelligence for Earth Observation) consolidates the pole position of Germany in AI4EO. The Lab serves multiple science fields: reasoning, uncertainties, explainable AI, physics-informed machine learning, complex structures, and more. It brings 20 renowned international organizations across 9 countries and 27 highly ranked scientists at all levels together to address fundamental challenges in Earth observation specific cutting-edge artificial intelligence research. The research carried out in the Future Lab AI4EO will not only advance Earth observation science but also make key contributions to the interpretability of AI, its ethical implications, and AI4EO technology transfer; the field of applications is also highly relevant to society. The Munich metropolitan region is one of the top places worldwide for AI education and research. The Lab itself is physically located at the new campus of TUM in Taufkirchen/Ottobrunn, where TUM is currently establishing its new Department of Aerospace and Geodesy as part of the Bavarian space initiative. The future lab AI4EO is also associated with the Munich Data Science Institute.
ML4Earth
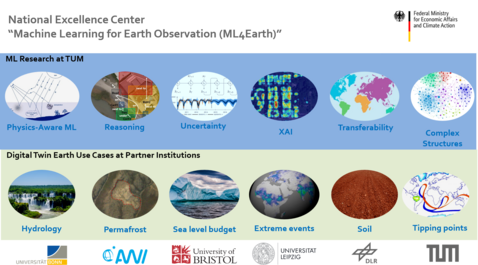
The national excellence center “Machine Learning for Earth Observation” (ML4Earth) will conduct own research at the highest international level by tackling fundamental methodical challenges in AI4EO and their application to the European mission of a Digital Twin Earth. ML research directions will include physics-aware machine learning, reasoning, uncertainty estimation, Explainable AI, Sparse Labels and Transferability, as well as Deep Learning for Complex Data Structures. By investing significant effort in advancing the community’s knowledge in these fields, we create direct impact in various application fields and are shaping our future globally. We will be able to assess the uncertainties of future sea level rise, make it more transparent and explainable how AI algorithms capture the increasing threat to global forests due to the increasing temperatures, quantify the inherent phenomenon of rapid permafrost thawing, use physical hydrological models paired with AI data science to predict Europe’s future ability to store water in cities given the increasing threat of extreme weather, map out physical and chemical soil parameters at large scale based on sparse knowledge on the soil parameters of quite localized areas, or apply deep learning to comprehend the complexity behind climate tipping points.
EarthCare: Twin Earth Methodologies for Biodiversity, Natural Hazards, and Urbanisation
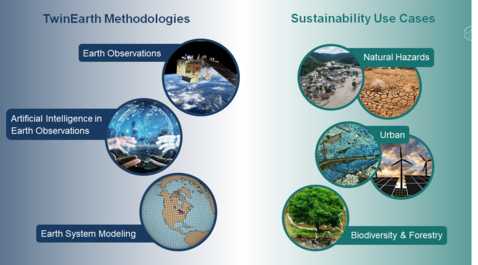
Anthropogenic climate changes pose severe threats to the Earth system, its ecosystems and human society. Effective strategies must be developed to mitigate further adverse changes and adapt to inevitable impacts, particularly the risks arising from an increase in extreme events. In doing so, transformation pathways toward sustainable Earth stewardship urgently need to be identified from global to local scales. The TUM Innovation Network EarthCare is an explorative and interdisciplinary study that bridges the knowledge gap between the use of Artificial Intelligence and Earth observation data for our planet’s sustainability. Under this general goal, EarthCare explores three main directions 1). methodologies for retrieving geoinformation from massive amount of Earth observation data, 2). Methodologies for creating hybrid earth system models of compartments of the Earth by combining machine learning and Earth observation data, and 3). Methodologies and use cases of impact models for decision of sustainability action. The vision of EarthCare is to provide key methodologies for science and policy making for a sustainable future.
Our PI team consists of high profile experts from four disciplines: 1.) Earth observation, 2). AI and data science, 3). Earth system modeling, and 4). Sustainability, which has unique capabilities to unlock the potential of merging highly innovative methods in Earth observation, artificial intelligence, and Earth system modeling with applications in biodiversity and forestry, the urban domain, and climate-induced natural hazards. The cross-cutting framework of EarthCare promotes the exchange between key disciplines of climate science and sustainability, and consolidate the scattered expertise in the Munich area.
iMonitor: AI for Monitoring Changes and Food Supply from Space

With the launch of the Sentinel satellite missions, the European Copernicus program has made freely available, an unprecedented volume of Earth observation data. These data provide information of the covered area in different spectral bands and at different times. The former is used to identify vegetated areas via the characteristic reflectivity of plants, the latter allows researchers to observe changes over time – a valuable source of information in times of rapid climate change and its consequences for food security. However, due to the complex nature of Earth observation data, current research is still only beginning to understand the opportunities created by analyzing the huge volume of data. This project is pushing forward the development of Artificial Intelligence methods that are inevitable in that regard. With iMonitor, we are focusing on algorithms that utilize the vast information behind multi-temporal data. The goal is to detect changes especially in agricultural areas at large spatial scales, unimpeded by cultural and geospatial differences of the land surface, only based on a few localized reference areas with well-known characteristics. Ultimately, we will be able to provide an important data source to quantify the fragility of the food supply dynamics and the impact of single-crop practices during increased pressure on soil, water availability, and drought.
OSMSim: OpenStreetMap Boosting using Simulation-Based Remote Sensing Data Fusion
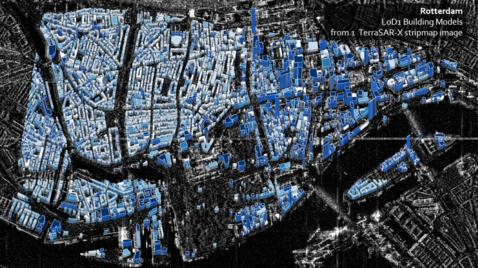
The main subject of the scientific investigations in this project is the improvement of building information (geometry, attributes) in OpenStreetMap (OSM) using a simulation-based fusion of heterogeneous remote sensing data and to use the updated OSM data for follow-up applications. The working basis is the simulation environment SimGeoI, which enables the modeling of imaging processes of different sensors by exploiting the metadata of remote sensing acquisitions as well as available geometric prior knowledge of the scene under investigation. SimGeoI allows not only a coarse semantic interpretation of the scene, but also an object-related alignment of corresponding scene elements. In this project, SimGeoI is used to compare geometric OSM information with remote sensing data produced under different sensor configurations and at different acquisition times, and to enrich OSM with geometric corrections (position, height) and attributes (e.g. building type, roof structure) gained from a fusion of the different remote sensing data. The scientific investigations of this project aim at three core themes: First, a methodical framework will be developed, which allows the geometric correction of OpenStreetMap data based on the prediction and comparison of building shapes, using a pair of remote sensing images (optical, SAR or mixed). In a second step, geometrically improved OSM information will be used to extract building-related attributes from multi-modal remote sensing data. Finally, the transferability of the developed methods will be experimentally analyzed and interfaces to follow-up applications (Open Event Mapping, Climate Event Portal, Virtual Reality) will be investigated. The methodology will be accompanied by validation in order to evaluate the positional, thematic and temporal accuracy of derived results.
Inno_MAUS – Innovative Instrumente zum Management des Urbanen Starkregenrisikos
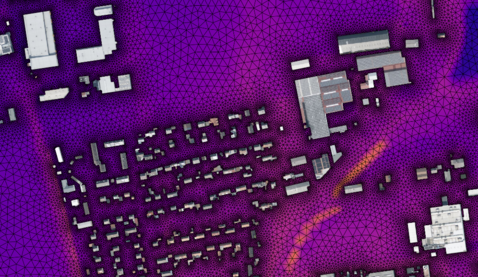
Extreme rainfall events pose a common problem that municipalities need to deal with. Due to the increased probability of extreme weather events due to Climate Change, however, immediate action is key to prevent massive damage or to mitigate risks. We respond to this challenge by facilitating cutting-edge data science to manage the risks going along with heavy rainfall events in a holistic fashion. We develop and train machine learning algorithms to process precipitation data in combination with remote-sensing information on the topography and urban morphology, to quantify very generally the effectiveness of drainage and water retention in the urban environment.
HGF W3: Data Science in Earth Observation – Big Data Fusion for Urban Research
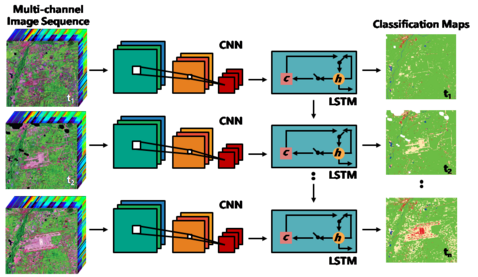
By 2050, around three quarters of the world’s population will live in cities. The new dimension of ongoing global urbanization poses fundamental challenges to our societies across the globe. Despite of increasing efforts, global urban mapping still drags behind the geometric, thematic and temporal resolutions of geo-information that would be needed to address these challenges.
Recently, big Earth observation data amounts in the order of tens of Petabytes (PBs) from complementary data sources have become available. For example, Earth observation (EO) satellites of space agencies reliably provide geodetically accurate large scale geo-information of worldwide cities on a routine basis from space. But the data availability is limited in resolution and viewing geometry. On the other hand, constellations of small and less expensive satellites owned by commercial players, like “Planet”, have been providing images for global coverage on a daily basis since 2017, yet with reduced geometric and radiometric accuracy.
As complementary sources of geo-information massive imagery, text messages and GIS data from open platforms and social media form a temporally quasi-seamless, spatially multi-perspective stream, but with unknown and diverse quality.
This project aims at jointly exploiting big data from social media and satellite observations for urban science.
Deep Transfer Learning in Remote Sensing
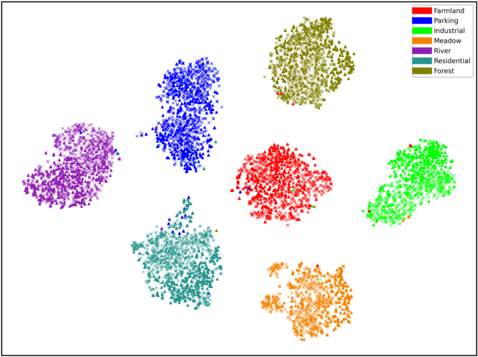
This project addresses knowledge transfer in Remote Sensing (RS) from an annotation-rich source domain to an annotation-scarce target domain by reducing their semantic discrepancy (domain shift), helping the latter and its follow-up applications without the need of numerous manually-labeled data. Specifically, the goal of the project is to design a universal deep Transfer Learning (TL) framework for RS data, named as deep RS-TL framework. Within this framework, on the one hand, we will develop several core deep TL algorithms to tackle several fundamental challenges of transferring knowledge in remote sensing, including source-target alignment, multi-temporal adaptation, multi-source adaptation, multi-scale adaptation, spatial-spectral adaptation, cross-task TL, cross-modality TL between source and target domain. On the other hand, we will construct an intelligent RS imagery annotation software which integrates all developed algorithms, to achieve flexible, personal and intelligent annotation for more efficient RS label collection. As a result, the anticipated deep RS-TL framework will considerably facilitate the practical applications of machine learning in remote sensing by relaxing its heavy dependence on laboriously labeled data.
EKAPEx: Energy-efficient AI for extreme weather events forecasting
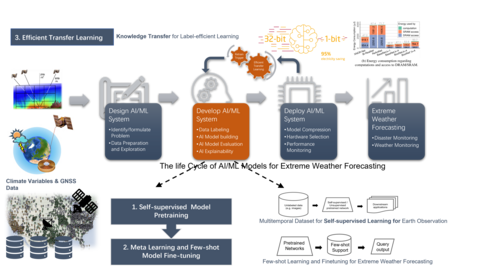
The project goal is AI-based precipitation forecast for Germany with a particular focus on extreme weather events. In this project, a novel method for precipitation forecast based on GNSS data and the traditional meteorological observation data. More specifically, two types of resource efficient AI methods will be developed, including label efficient AI methods and computation efficient AI methods.
Horizon Europe – MultiMiner
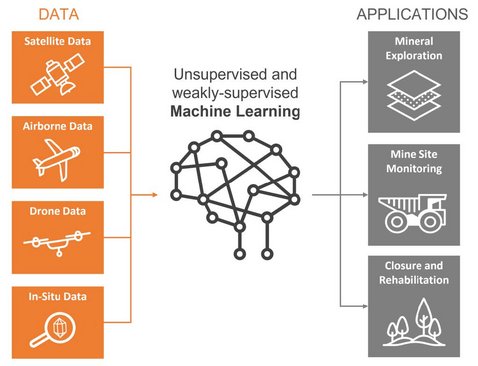
The European Union (EU) is dependent on imports of raw materials and is susceptible to disruptions caused by various factors such as war, pandemics, changes in China's power supply, and shifts in US monetary policy. To increase its strategic autonomy, the EU aims to increase domestic production through successful mineral exploration projects. At the same time, the EU Green Deal sets a goal of a sustainable, carbon-neutral EU economy by 2050. To achieve these objectives, the entire mining life cycle must adopt minimal-impact exploration and monitoring technologies that provide accurate and reliable data.
Current methods of earth observation (EO) data analysis and interpretation in the mining industry are heavily reliant on expert knowledge and in situ data, which can disrupt mining activities and reduce cost-efficiency. The MultiMiner project seeks to address this challenge by developing novel data processing algorithms for efficient utilization of EO technologies in mineral exploration and mine site monitoring. This project focuses on developing a multi-scale, weakly supervised/unsupervised approach for detecting minerals of interest, allowing for semi-automated interpretation of remotely sensed data by experts and non-experts. The algorithm will process available data sources at multiple spatial, temporal, and spectral resolutions and can be easily adapted for various monitoring tasks, including vegetation, Acid Mine Drainage (AMD), water quality, ground stability, ground moisture, and dust, throughout the entire mining life cycle.
The MultiMiner project prioritizes the development of EO-based exploration technologies for critical raw materials (CRM) to increase the likelihood of finding new sources within the EU and enhance the EU's autonomy in the raw materials sector. The project's EO-based exploration solutions have a low environmental impact and are socially acceptable, economically efficient, and improve safety. Additionally, the solutions for mine site monitoring increase transparency in mining operations by detecting environmental impacts as early as possible and preserving digital information for future generations.
AI4TWINNING
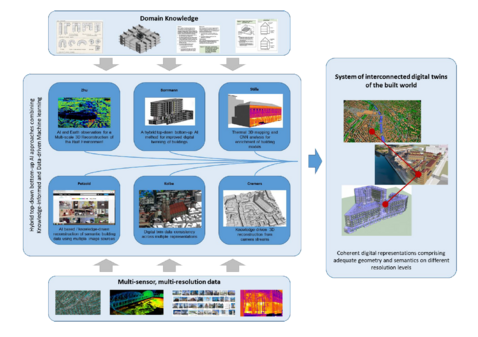
Recently big Earth observation data amounts in the order of tens of Petabytes from complementary data sources have become available. For example, Earth observation (EO) satellites reliably provide large scale geo-information of worldwide cities on a routine basis from space. However, the data availability is limited in resolution and viewing geometry. On the other hand, closer-range Earth observation platforms such as aircrafts offer more flexibility on capturing Earth features on demand. This sub-project is to develop new data science and machine learning approaches tailored to an optimal exploitation of big geospatial data as provided by abovementioned Earth observation platforms in order to provide invaluable information for a better understanding of the built world. In particular, we focus on the 3D reconstruction of the built environment on an individual building level. This research landscape comprises both a large scale reconstruction of built facilities, which aims at a comprehensive, large scale 3D mapping from monocular remote sensing imagery that is complementary to those derived from camera streams (Project Cremers), as well as a local perspective, which aims at a more detailed view of selected points of interest in very high resolution, which can serve as basis for thermal mapping (Project Stilla), semantic understanding (Project Kolbe & Petzold) and BIMs (Project Borrmann). From the AI methodology perspective, while the large-scale stream will put the focus on the robustness and transferability of the deep learning/machine learning models to be developed, for the very high-resolution stream we will particularly research on the fusion of multisensory data, as well as hybrid models combing model-based signal processing methods and data-driven machine learning models for an improved information retrieval. With the experience gained in this sub-project, we will lay the foundation for future Earth observation that will be characterized by an ever-improved trade-off between high coverage and simultaneously high spatial and temporal resolutions, finally leading to the capability of using AI and Earth observation to provide a multi-scale 3D view of our built environment.
Deep Learning for Domain Specific Object Detection in Satellite Images
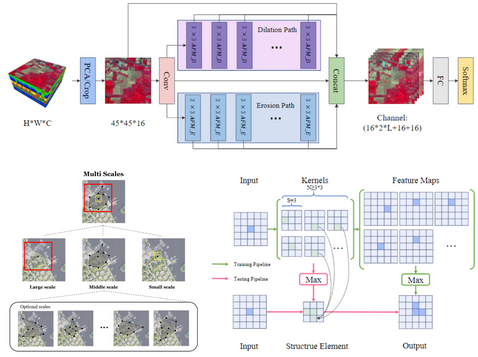
The past decade has witnessed major advances in deep learning for object detection in vision tasks. However, the flourish of deep learning is mainly accredited to the rich annotated data. The gaps between experimental environment and realistic scenarios result in catastrophic performance degradation when the algorithms are deployed in practical satellite images. This project focuses on the object detection in the domain specific scenarios, which faces the noteworthy and typical real-world applications with environmental constraints. Two main issues in this research field are eager to be tackled. The first one is how to extract robust deep features with insufficient and partially annotated training set, i.e., weakly supervised deep learning for object detection on satellite image. The second is how to detect objects of novel categories with only a few given examples, i.e., few-shot object detection.
Finished Projects
ERC StG So2Sat: 10^16 Bytes from Social Media to Earth Observation Satellites
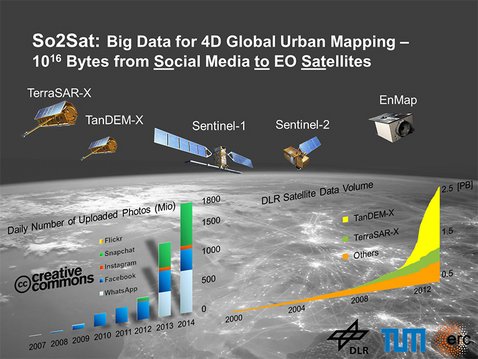
So2Sat is an ambitious European Research Council (ERC) starting grand project. In the project, we will use revolutionary mapmaking methods to investigate how human settlements grow. We have the previlege to access the data supplied by several German and European earth observation satellites, which are equipped with innovative sensor technologies. We will develop new algorithms for the derivation of geo-information from these measurements. This makes it possible to create high-resolution 3D/4D maps of the cities up to individual building. For the first time, this information will also be combined with data from social networks: crowdsourcing platforms such as OpenStreetMap providing up-to-date map material; photos posted to the network providing authentic and current images in which buildings can be seen or which for example reveal the extent of damage caused by a flood. The major challenge here is consolidating this information and evaluating it automatically in a global scale.
AI4Sentinels
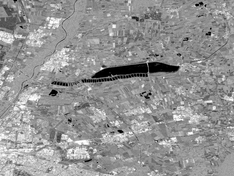

The Sentinel 1 and Sentinel 2 missions outperform comparable satellite missions; the Sentinel satellites are designed to observe the entire Earth’s surface at higher cadences, with higher spatial resolution, and by adding sensors scanning at radar wavelengths. These advantages also come with challenges. First, the figure shows how fundamentally different the information content is for images of both missions, which observe the same region of Munich at radar and optical wavelengths, respectively. On top, all mentioned advantages of the Sentinel missions result in a vastly increased total data volume. The community therefore had to investigate innovative approaches to effectively process these data for large-scale monitoring projects. We have responded to this challenge with the project AI4Sentinels. It turns out that Artificial Intelligence (AI) techniques help to process these diverse data and exploit maximal information out of the imagery.
Stereogrammetric Fusion of Optical and SAR Data for the Reconstruction of Urban Scenes
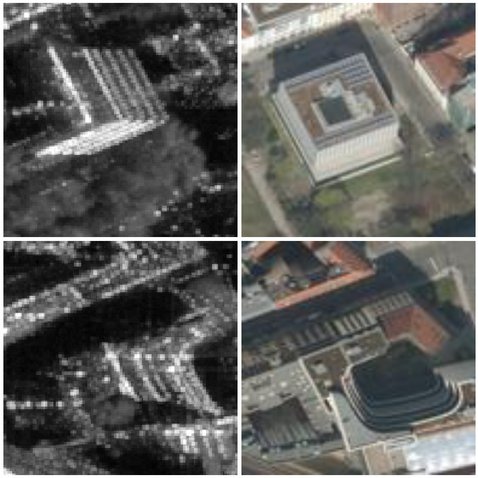
The main subject of the scientific investigations within this project is the reconstruction of urban scenes by a stereogrammetric fusion of high-resolution spaceborne optical and SAR image data. The goal of this kind of sensor data fusion is to get a comprehensive three- dimensional description of urban topography. There are several reasons for this fusion: On the one hand, particularly spaceborne optical imagery are widely available and stored in great amounts in international Earth observation archives. On the other hand, also recent radar remote sensing missions, such as TerraSAR-X/TanDEM- X or CosmoSkymed, lead to a growing availability of high-resolution SAR data. Making use of the possibility to acquire new SAR data independently from daylight or weather conditions, this project wants to support the request to exploit existing archive data of regions of interest with greater flexibility but also to enable a timely mapping of critical areas by optimum combination of arbitrary satellite image data that is available on short notice. The results of this project will help to make the analysis of heterogeneous satellite image data as flexible as possible, in particular with respect to a rapid 3D mapping in time- critical applications.
Multisensory Data Fusion for Land Cover Mapping
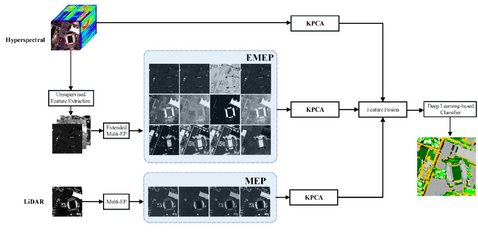
The increased availability of data from different satellite and airborne sensors for a particular scene makes it desirable to jointly use data from multiple data sources for improved information extraction, hazard monitoring, and land cover/land use mapping. In this context, hyperspectral sensors provide detailed spectral information, which can be used to discriminate different classes of interest, but they do not provide structural and elevation information. On the other hand, LiDAR data can extract useful information related to the size, structure, and elevation of different objects, but cannot model the spectral characteristics of different materials. The main objective of this project goes to the proposition of efficient approaches for the integration of LiDAR and hyperspectral data.
Coupled Spectral Unmixing for Multidimensional High-Resolution Analysis
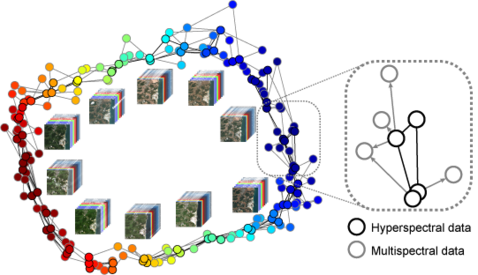
The objective of the project is to develop a methodological framework for generalized coupled spectral unmixing to simultaneously unmix multisensor and multitemporal spectral images. The framework is applied to time series analysis and resolution enhancement of hyperspectral imagery. The outcome of the project will promote synergy and fusion of spaceborne hyperspectral and multispectral data (e.g., EnMAP and Sentinel-2).
Large-Scale Problems in Earth Observation
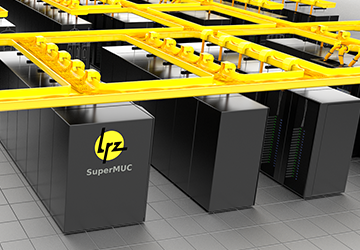
Satellite remote sensing enables us to recover contact-free large-scale information about the physical properties of our Earth system from space. For information retrieval from these massive Earth observation data, efficient computing is necessary. To develop faster algorithms, especially for those large-scale problems that arise in Earth observation, it is thus inevitable to consider parallel computing. To this end, an interdisciplinary approach including optimal information retrieval and computationally efficient, parallelized solvers for large-scale problems seems the optimal solution, which is the focus of this project. Figure by courtesy of LRZ.
SiPEO: Signal Processing in Earth Observation
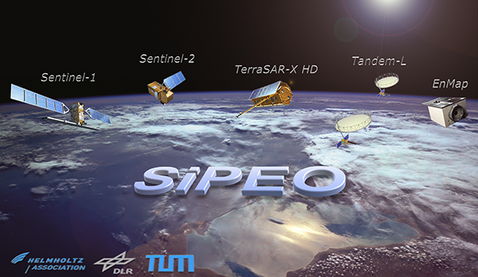
SiPEO develops explorative algorithms to improve information retrieval from remote sensing data, in particular those from current and the next generation of Earth observation missions. Currently, the team is working on the following main areas: 1) sparse Earth observation; 2) non-local filtering concept; 3) robust estimation. The improved retrieval of geo-information from EO data can be used to better support cartographic applications, resource management, civil security, disaster management, planning and decision making.
SparsEO: Sparse Reconstruction and Compressive Sensing for Remote Sensing and Earth Observation
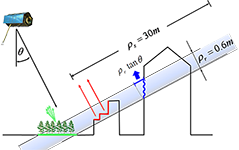
This projects exploits the sparsity in remote sensing data, e.g. SAR signal in the elevation direction, and LiDAR full waveform. The project will identify sparse signals in remote sensing data, proide forward modeling and inversion techniques. Fast parallel sparse reconstruction solvers tailored to our problems will also be developed.
4D City: Spatial-temporal Reconstruction of City Models from Meter-resolution SAR Data
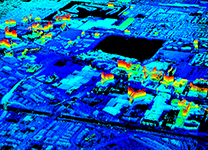
The research envisioned in this project leads to a new kind of city models for monitoring and visualization of the dynamics of urban infrastructure in a very high level of detail. The change or deformation of different parts of individual buildings will be accessible for different types of users (geologists, civil engineers, decision makers, etc.) to support city monitoring and management as well as risk assessment.
CSThz: Compressed Sensing for Terahertz Body Scanners
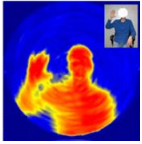
The objective of the project is to develop Compressive Sensing reconstruction algorithms for terahertz (THz) body scanners in order to improve the image quality of these scanners. Our project part focuses in particular on the development of joint 3D reconstruction techniques for FMCW THz radar imaging, that combine THz imaging in x,y and FMCW radar in the z direction. Using regularizers and exploiting the sparse properties of the signal during reconstruction, we attempt to improve quality and acquisition speed. Figure by courtesy of Sven Augustin.
Object Reconstruction Using TomoSAR Point Clouds
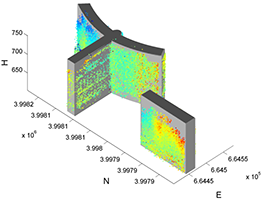
This project is a follow-up of the project "4D City". It attempts the first reconstruction of objects from 3-D tomographic SAR point clouds, with the vision of building dynamic city models that could potentially be used to monitor and visualize the dynamics of urban infrastructure in very high level of details. The basic idea is to reconstruct 3-D building models via independent modeling of each individual façade to build the overall 2-D shape of the building footprint followed by its representation in 3-D.
J-Lo: The Joy of Long Baseline
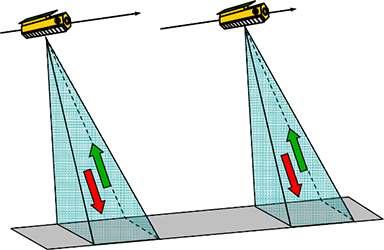
This project makes use of the special configurations of the TanDEM-X Science Phase for precise 3D point localization and coastline detection. A joint feature of the investigated applications is the exploitation of large spatial and temporal baselines, which are available in Pursuit Monostatic Mode during the Science Phase. In this phase also the relatively new, high resolution Staring Spotlight Mode will be available for the first time in a single-pass interferometric configuration.