Deep Transfer Learning in Remote Sensing
Funded by the DFG
PI
Xiaoxiang Zhu
Project Scientists
Wei Huang
This project addresses knowledge transfer in Remote Sensing (RS) from an annotation-rich source domain to an annotation-scarce target domain by reducing their semantic discrepancy (domain shift), helping the latter and its follow-up applications without the need of numerous manually-labeled data. Specifically, the goal of the project is to design a universal deep Transfer Learning (TL) framework for RS data, named as deep RS-TL framework. Within this framework, on the one hand, we will develop several core deep TL algorithms to tackle several fundamental challenges of transferring knowledge in remote sensing, including source-target alignment, multi-temporal adaptation, multi-source adaptation, multi-scale adaptation, spatial-spectral adaptation, cross-task TL, cross-modality TL between source and target domain. On the other hand, we will construct an intelligent RS imagery annotation software which integrates all developed algorithms, to achieve flexible, personal and intelligent annotation for more efficient RS label collection. As a result, the anticipated deep RS-TL framework will considerably facilitate the practical applications of machine learning in remote sensing by relaxing its heavy dependence on laboriously labeled data.
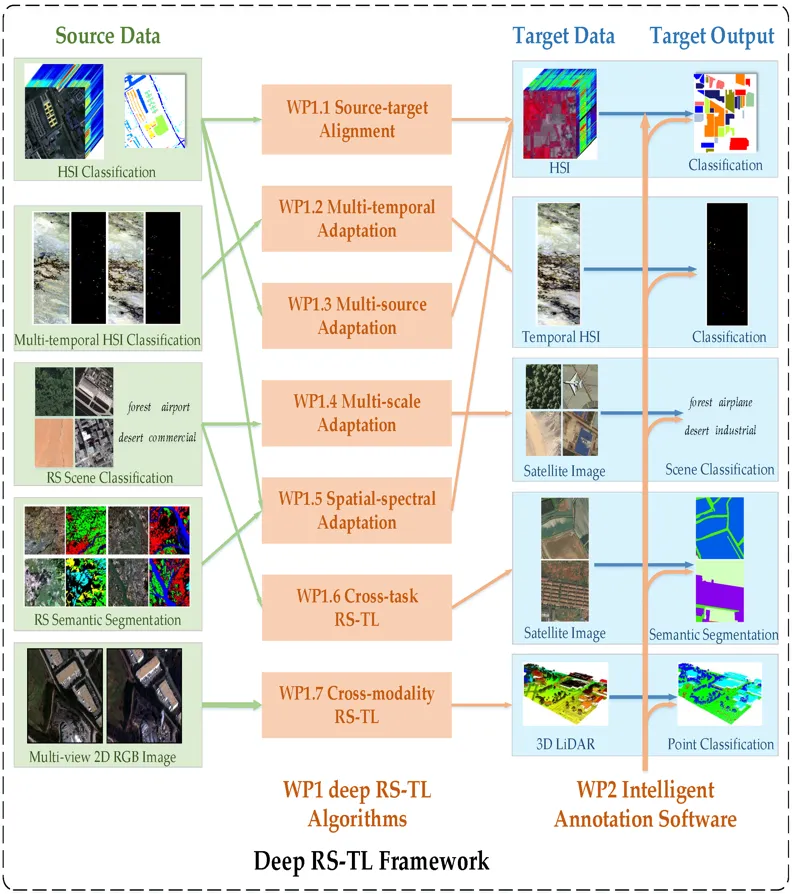